Leveraging Privacy Enhancing Technologies to Support UK Life Sciences
-
Using PETs to Drive AI/ML
The application of artificial intelligence and machine learning (AI/ML) to healthcare domains, including drug discovery and clinical diagnostics, is increasingly promising, as these technologies can recognise complex patterns in large volumes of health data. However, accessing high quality health data – particularly demographically representative data—remains a significant challenge for organisations implementing data-driven innovations. As AI/ML transforms life sciences, accelerating access to health data can help research organisations develop medicines and medical devices with a higher probability of success, and increasing access to representative data can ensure that the benefits of AI/ML in healthcare are shared across demographic groups.
Privacy Enhancing Technologies (PETs) provide a partial solution by increasing access to sensitive health data whilst safeguarding patients’ right to privacy. The UK is uniquely positioned to leverage PETs to support AI/ML adoption in its life sciences industry, in line with the ambitions of its AI Opportunities Action Plan.
-
What are PETs?
PETs are a collection of tools that enable access to data that would otherwise be inaccessible for privacy reasons. These tools include synthetic data, federated learning and trusted research environments (TREs):
-
Synthetic data: artificially generated to reproduce key statistical or structural properties of real data, aiming to preserve the privacy of original data subjects by preventing re-identification. Synthetic data can support AI/ML development and training without exposing sensitive personal information, enabling innovation while maintaining compliance with data protection regulations.
-
Federated learning: a decentralised approach in which model training is distributed across multiple nodes or institutions, and only model updates (e.g., gradients or weights) are shared—not the underlying data. Federated learning can facilitate collaboration on model development across institutional boundaries without centralising sensitive data, reducing privacy risks while leveraging diverse datasets for improved model performance.
-
Trusted research environments (TREs): secure computing environments providing remote access to data. TREs enable analysis of sensitive datasets within a controlled setting with built-in security measures, governance protocols, and audit trails to ensure appropriate data usage while maintaining privacy protections.
Synthetic data, federated learning, and TREs are complementary and can be combined: for example, federated learning may occur within or across TREs, and synthetic data may be used to simulate or prototype analyses before secure access.
-
How are PETs being used?
PETs are used across sectors to help solve real-world data access challenges, whilst protecting data privacy. In the UK, openSAFELY’s platform enables researchers to analyse millions of NHS primary care records without direct access, using federated analytics within secure environments. Researchers are provided with low fidelity ‘dummy’ data to develop their own analytical code, which is then run by openSAFELY on real data with federated analytics to generate insights from across the primary care system. This is designed for data privacy so that researchers never interact directly with patient records.
Outside of the UK, IDERHA (Integration of Heterogeneous Data and Evidence towards Regulatory and HTA Acceptance) is one of the first research projects funded through a public-private partnership between the European Union (EU) and European life sciences industries. IDERHA seeks to build a pan-European health data space to facilitate access and analysis of diverse health data. Similarly to openSAFELY, IDERHA aims to leverage federated approaches to enable decentralised insights from data.
These use cases highlight a shift in the health data landscape where PETs are increasingly seen as serviceable tools for unlocking health data whilst respecting patient data rights.
-
What are the benefits of PETs?
There are numerous use cases in which PETs can accelerate biopharmaceutical research. Consider key limiting factors in the implementation of machine learning in biopharmaceutical organisations;
-
the rate at which organisations can ingest health data
-
assess the utility of the data
-
request different sets or subsets of data if the initial data is not as useful as expected
-
design the appropriate model architecture to train on the data
All of the above can act as barriers to ML training. However, if organisations have clearer indications of the content of a dataset, they’re able to choose which data to ingest more carefully and to prepare their models well before they ingest the data, saving them weeks and months at a time. This principle is already at work in openSAFELY, but more access to greater fidelity synthetic data prior to ingestion would be an impactful accelerant for biopharma research.
The use of PETs to access health data is of particular benefit to the NHS given the nationally funded model of health provision in the UK. As the primary provider of healthcare, the NHS stores a wealth of health data that spans across lifetimes, and also systems (e.g. primary and secondary care). The data created through the NHS’ continuity of care has huge potential to provide insights into health, that other fragmented health systems may fail to capture. As well as this, the national health coverage provided by the NHS not only leads to greater data availability, but also data diversity – given the UK’s relatively diverse population—which is necessary for training equitable and generalisable models across socioeconomic groups, ethnicities, and regions.
-
Considerations when using PETs
PETs require risk-based governance and technical controls to ensure robust and responsible use. Systematic frameworks that ensure transparent use of data, respect patient consent and privacy, and prevent misuse, are necessary to avoid failures of public trust that have historically harmed efforts to open health data in the UK.
Similarly, as they are doing in developing general technical standards for AI/ML, policymakers can work with industry practitioners to establish best practice technical standards for the use of synthetic data and other PETs that ensure robust and reliable performance and avoid model collapse, overfitting, and other pitfalls in the improper use of PETs. Absent these controls, the potential for privacy violations and disappointing model performance risk diminishing confidence in these technologies.
There are several public policy questions to resolve in developing clear governance norms. This includes, in some cases, striking an appropriate balance between utility and privacy: for example, synthetic data can vary in its fidelity to the original data; ceteris paribus, higher fidelity synthetic data is more useful because it more closely approximates the actual data, but this increases the risk that sensitive patterns, outliers, or individual records could be inferred or re-identified from the synthetic data.
DSIT and the ICO have already developed useful tools to guide organisations in using PETs. Further government guidance can help clarify for health bodies and research organisations how to navigate this trade-off and create standards for patient consent, transparency, and other ethical safeguards.
-
Seizing the Opportunity
Although the use of PETs has gained traction in recent years, further clinical validation is needed in the health data space. The NHS is currently undergoing an artificial data pilot exploring the utility of low fidelity synthetic data. Further work around synthetic data, particularly exploration of the use cases, benefits and limitation of higher fidelity synthetic data are of particular interest. Although federated analytics is currently being used in the NHS, pilots of federated training of machine learning models are still yet to be fully explored.
We believe that much of the reticence surrounding PETs stems from the absence of policy frameworks around their use. Regulators and policymakers can seize the opportunity by issuing clearer guidance on the use of PETs to cultivate certainty and confidence. This is exemplified by forward-thinking governments like Singapore who have issued guidance to take advantage this technology. Whilst the UK Government is searching for AI opportunities, we suggest that expanding PETs is a potentially high-reward opportunity for one of the UK’s leading sectors.
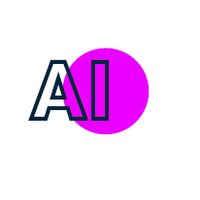
techUK - Seizing the AI Opportunity
For the UK to fully seize the AI opportunity, citizens and businesses must have trust and confidence in AI. techUK and our members champion the development of reliable and safe AI systems that align with the UK’s ethical principles.
AI assurance is central to this mission. Our members engage directly with policy makers, regulators, and industry leaders to influence policy and standards on AI safety and ethics, contributing to a responsible innovation environment. Through these efforts, we help build public trust in AI adoption whilst ensuring our members stay ahead of regulatory developments.
Get involved: techUK runs a busy calendar of activities including events, reports, and insights to demonstrate some of the most significant AI opportunities for the UK. Our AI Hub is where you will find details of all upcoming activity. We also send a monthly AI newsletter which you can subscribe to here.
Upcoming AI events
Latest news and insights
Subscribe to our AI newsletter
AI and Data Analytics updates
Sign-up to our monthly newsletter to get the latest updates and opportunities from our AI and Data Analytics Programme straight to your inbox.
Contact the team
Visit our AI Hub - the home of all our AI content:
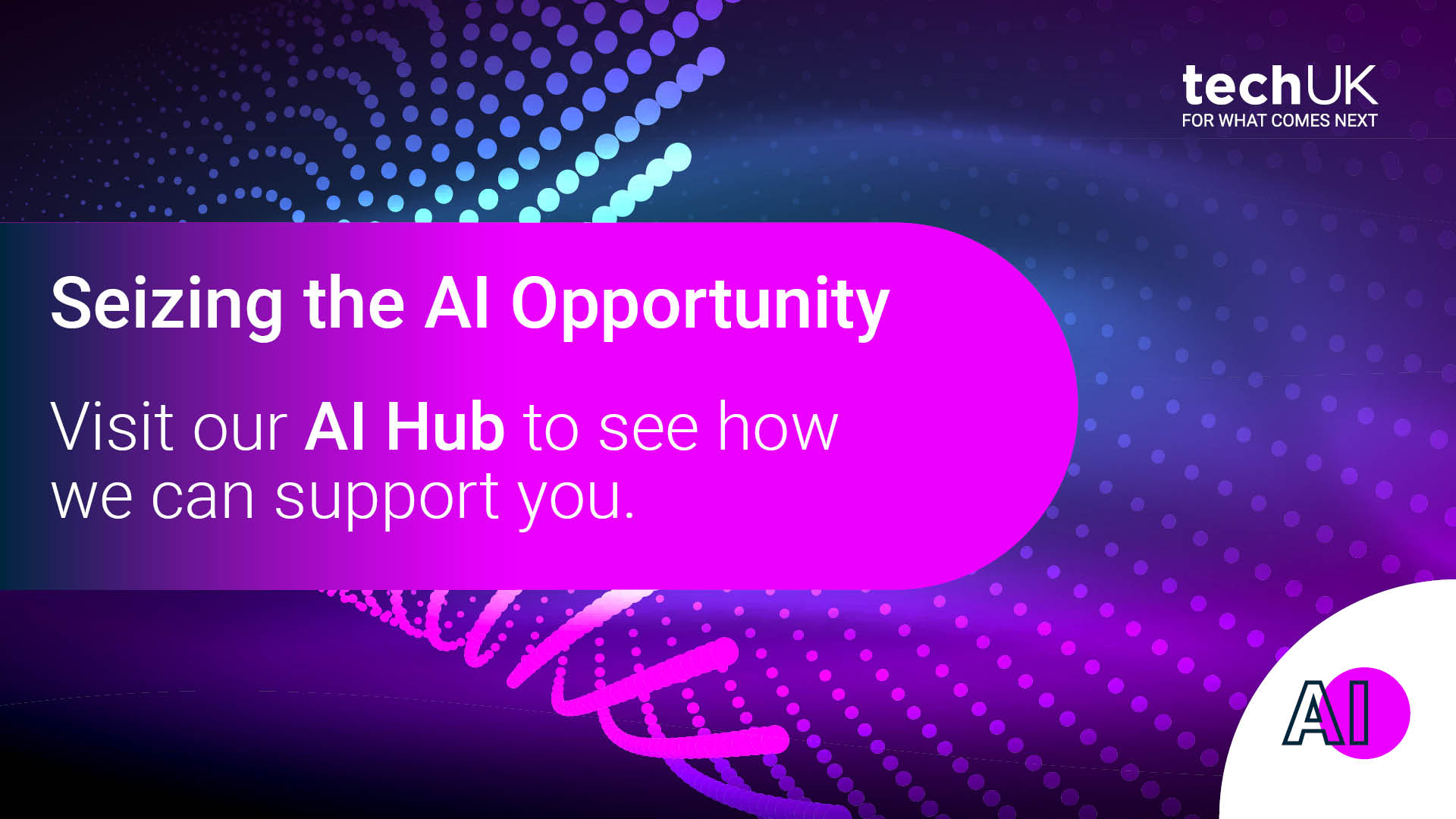