Event round-up: Putting AI into Action webinar on making data work for businesses
Chair:
- Usman Ikhlaq - Programme Manager for Artificial Intelligence, techUK
Panellists:
- Emma Erskine-Fox – Managing Associate, TLT
- Bharat Bhushan - Chief Technology Officer, KPMG Consulting
- Simon de Timary – Head of Data & AI, BJSS
- Sam Lloyd – Head of Data, Ocado Technology
Recording:
Summary:
1. What does successful data look like for businesses?
- Balance: Harnessing data for maximum impact while adhering to legal and regulatory requirements, which should act as enablers, not barriers, to innovation.
- Scalability: Data systems must support large-scale operations without compromising performance. Proof-of-concept solutions often work on a small scale, but successful data means scaling up effectively while maintaining access and functionality.
- Organisational excellence: Data should drive better decision-making, enhance operations, and integrate AI as a priority at the executive level for business success.
2. Why is data (including quality and governance) important for AI?
- Data quality: The principle of "garbage in, garbage out" underscores the importance of input data quality. Even accurate data can lead to biased outputs if not carefully selected. For example, algorithms for predicting criminal recidivism have inadvertently introduced bias via proxies like postcodes.
- Governance: Beyond data governance, a wider governance framework is critical. For example, general AI and voice recognition tools require monitoring for potential sentiment analysis biases.
- Trust and accuracy: In industries like finance or healthcare, inaccurate data can lead to severe consequences. Building systems that ensure the trustworthiness, accuracy, and scalability of data is essential.
- Efficiency: Currently, 80% of analysts' time is spent sourcing, cleaning, and understanding data lineage, leaving only 10% for value-added work. Improving data processes and democratizing access can boost effectiveness.
3. What are the key data challenges businesses face, and how do they stop AI adoption?
- Operational data management: Data lakes and operational data stores can lead to performance issues and scalability limitations. Managing global datasets while adhering to compliance rules adds complexity.
- Legal and compliance barriers: Legal teams often lack clear frameworks to evaluate AI tools, creating bottlenecks. Streamlining processes and frameworks can help organisations meet their AI objectives.
- Silos: Mergers and fragmented data storage create impenetrable silos. This makes it difficult to consolidate data from different sources (e.g., sales, stock, marketing) to power AI models.
- Cultural resistance: A lack of integration between business strategy and technical implementation creates hurdles. Cultural shifts are needed to foster AI adoption and bridge commercial and technical understanding.
- Regulatory certainty: The evolving AI landscape requires clear, consistent rules. Engaging with regulators, such as the ICO or EU bodies, can help businesses address concerns and ensure compliance.
4. What can organisations and the UK Government do to resolve these issues?
To address data quality and governance challenges:
- Enable data sharing: The government can establish standards for data presentation and create secure marketplaces to facilitate inter-agency and public-private data sharing.
- Leverage funding: The apprenticeship levy can upskill staff, combining domain expertise with AI and data analytics knowledge. This can unlock the potential of existing workforces.
- Collaborate across sectors: Bringing together industries, consumer groups, and academics can foster innovation, build energy-efficient models, and improve governance. The UK’s leadership in open banking standards serves as a model for other sectors.
- Streamline governance: Clear guidelines and streamlined frameworks for data governance and compliance can reduce roadblocks, enabling quicker AI adoption while maintaining security and trust.
Key takeaways for businesses:
- Invest strategically: Treat data quality and governance as strategic investments rather than overheads.
- Adopt simplicity: Begin with simple AI solutions, iterating and scaling as needed.
- Be collaborative: Emphasise collaboration across internal teams, sectors, and regulators to drive innovation.
- Set clear goals: Ensure AI solutions address specific business needs, focusing on use cases rather than technology for its own sake.
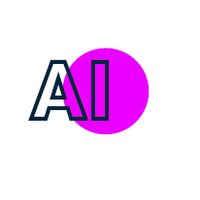
techUK - Seizing the AI Opportunity
The UK is a global leader in AI innovation, development and adoption.
AI has the potential to boost UK GDP by £550 billion by 2035, making adoption an urgent economic priority. techUK and our members are committed to working with the Government to turn the AI Opportunities Action Plan into reality. Together we can ensure the UK seizes the opportunities presented by AI technology and continues to be a world leader in AI development.
Get involved: techUK runs a busy calendar of activities including events, reports, and insights to demonstrate some of the most significant AI opportunities for the UK. Our AI Hub is where you will find details of all upcoming activity. We also send a monthly AI newsletter which you can subscribe to here.
Upcoming AI events
Latest news and insights
Subscribe to our AI newsletter
AI and Data Analytics updates
Sign-up to our monthly newsletter to get the latest updates and opportunities from our AI and Data Analytics Programme straight to your inbox.
Contact the team
Visit our AI Hub - the home of all our AI content:
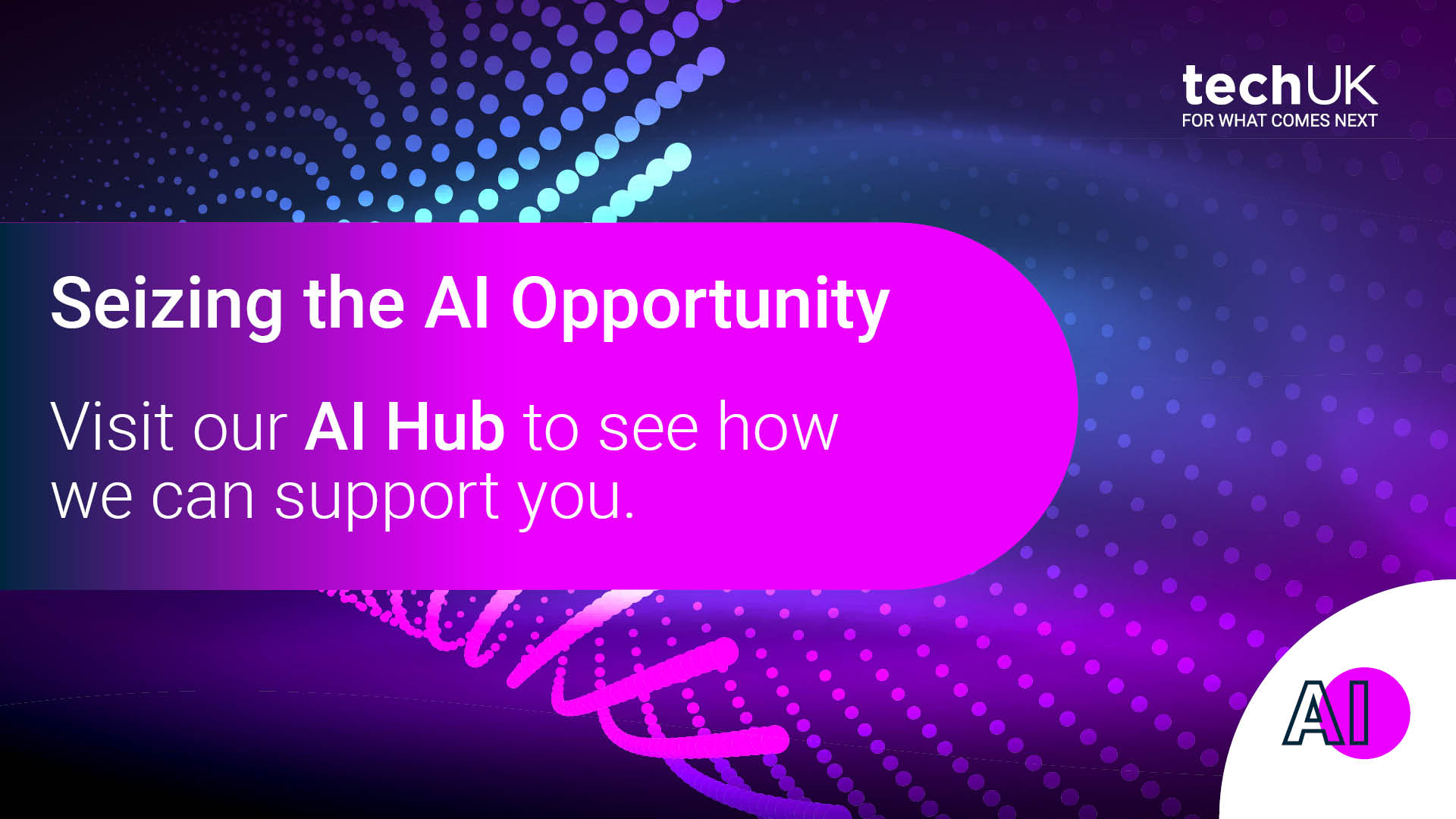