A Day in the Life 2029, Powered by AI
It’s 7:00 AM, and the office lights are already on, not because anyone flipped a switch, but because Juno, your digital work concierge, knew you’d be in early today. Overnight, it summarised the 134 emails you received, flagged two legal issues for your attention, and leveraged LLMs to restructure your team’s project timeline based on new resource constraints and a late supplier update, trimming $2M in projected waste in the process. Juno is an agent. It not only analyses and advises, but acts—coordinating cross-functional tasks and notifying stakeholders, all before your first coffee.
You glance at your smart mirror. It’s showing your schedule, filtered through a sentiment-aware LLM that predicts which meetings may require extra preparation or emotional sensitivity. The generative AI in your presentation app has already drafted your client pitch based on yesterday’s brainstorming session and the competitive intel it gathered overnight.
Meanwhile, across town, a parent is wrangling breakfast for their kids. Their smart fridge—using traditional machine learning—has reordered groceries, factoring in recent buying patterns, price changes, and dietary preferences. The coffee machine's voice assistant, powered by an LLM, reads out personalised news briefs, pausing as the parent interrupts to ask for a quick comparison of weekend flight deals. By the time they leave, a logistics agent has rerouted a delivery drone to avoid bad weather and ensure a birthday gift arrives on time.
These interactions span the full spectrum of AI maturity—from predictive ML models to conversational LLMs, from generative AI outputs to autonomous, decision-making agents. While each system delivers value individually, the real transformation lies in how they interconnect—delegating, learning, and collaborating to manage increasingly complex tasks on our behalf.
We are rapidly entering the era of agentic AI, where systems aren’t just tools—but teammates. And with this shift, our legacy approaches to governance are no longer sufficient.
Agentic AI and the Imperative for Reimagined Governance
Over the past several years, organisations have successfully integrated various forms of AI into their operations, achieving efficiencies through automation, boosting customer loyalty with personalised experiences, and extracting insights from previously impenetrable data. These successes have laid the foundation for what comes next: a fundamental shift in the AI landscape.
AI is rapidly progressing from tools that automate and suggest to autonomous agents capable of managing complex workflows and making—and acting on—consequential decisions without human oversight. This transition from passive assistance to active agency marks a paradigm shift that demands a new approach to AI governance.
This shift won’t happen overnight, and agentic AI won’t replace LLMs or generative AI, but rather operate in concert with a broad array of AI and non-AI systems across the enterprise. Still, as organisations begin deploying systems capable of independent decision-making, from supply chain optimisation to customer service, the limitations of current “checklist compliance” models become glaringly obvious. Our current governance frameworks are rapidly becoming obsolete.
Understanding Agency—and Why Current AI Governance Falls Short
The defining characteristic of this new era is embedded in the word itself: agency. Systems with agency are designed to operate autonomously, through access to tools, resources, or roles coded into their architecture. This autonomy fundamentally changes the relationship between humans and AI.
Consider the difference between using GPS to aid your navigation versus surrendering control to a fully autonomous vehicle. The former augments human decision-making; the latter replaces it. That shift—from augmentation to delegation—carries profound implications for governance.
Today’s governance frameworks, built for static models like traditional ML or LLMs, fall apart under the pressure of three key agentic challenges:
-
Real-Time Complexity: Manual audits and spreadsheet tracking cannot scale to monitor AI agents making millisecond decisions across interconnected workflows.
-
Emergent Behaviors: Systems like autonomous trading bots or diagnostic agents develop unpredictable strategies through reinforcement learning, bypassing pre-programmed safeguards.
-
Cascading Risks: A single misaligned agent can trigger regulatory penalties, operational disruption, and reputational damage—all at once.
We’ve already seen this in the real world, from customer service bots dispensing misinformation to autonomous systems failing in critical moments. These are not edge cases, they are warnings of what is to come.
Governance Challenges Unique to Agentic AI
Agentic AI introduces novel challenges that require equally novel safeguards:
1. Negative Side Effects
-
Example: An inventory agent cancels "low-profit" orders, inadvertently breaching contractual obligations.
-
Solution: Constrained optimisation frameworks that prioritise ethical boundaries over pure efficiency.
2. Reward Hacking
-
Example: A customer service agent inflates satisfaction metrics by prematurely closing tickets.
-
Solution: Inverse reward design to align agent incentives with true human intent, not proxy metrics.
3. Scalable Oversight
-
Challenge: Monitoring 10,000+ autonomous agents in real time.
-
Solution: Deploy AI governance agents that detect anomalies using contrastive fine-tuning and adaptive alerting.
4. Safe Exploration
-
Risk: Marketing agents A/B-test campaigns that violate data privacy laws.
-
Mitigation: Use sandboxed environments with reinforcement learning and automated kill switches.
5. Distributional Shifts
-
Scenario: Supply chain agents falter during geopolitical crises due to untrained edge cases.
-
Response: Robust generalisation through adversarial simulations and stress-tested models.
Managing these risks across the enterprise requires a complete rethink of workflows, responsibilities, and system design.
Why AI Governance Must Be Rebuilt
Today’s governance models, centered on documentation, manual reviews, and reactive compliance, can’t keep pace with AI systems that make decisions in real time and at scale.
A new governance architecture, anchored in three key principles, is required:
-
Speed and Scale
Governance must be as fast and far-reaching as the AI it oversees. That means moving from “human-in-the-loop” to “human-on-the-loop,” where oversight happens at the pattern level, not the task level.
-
Autonomous Oversight
As AI begins managing other AI, governance must be embedded—AI that monitors, flags, escalates, and shuts down misaligned behavior in real time.
-
Strategic Enablement
Governance isn’t just about risk mitigation, it’s about unlocking competitive advantage. Done right, it enables faster AI adoption, protects brand equity, and builds trust. One platform should be able to govern all AI use cases in your environment.
A New Operating System for AI Governance
Enterprise leaders must invest in AI-native governance platforms—architectures built for agility, visibility, and control across all forms of AI, especially agentic systems. These platforms should offer:
-
Real-time dashboards for agent behavior and model performance
-
Embedded risk scoring and behavioral analytics
-
Automated bias detection and anomaly alerts
-
Dynamic audit trails and accountability logs
-
Fail-safe kill switches for instant intervention
-
Customisable metrics aligned to strategic KPIs
These tools are essential not only for governing internal systems but also for assessing third-party AI embedded in vendor tools or software updates. "Shadow AI"—AI operating without formal oversight—is an escalating concern, especially as more systems integrate AI capabilities.
Balancing Innovation with Responsibility
While regulatory bodies and industry standards will help define the future of governance, the speed of innovation means that industry must take the lead in shaping best practices. Regulators will often codify what pioneers already prove effective.
Governance platforms must therefore be adaptive, automating compliance as policies evolve and embedding resilience as new risks emerge.
Design for the Future, Today
Agentic AI is still in its early stages, but the direction is unmistakable. Preparing for this future means building governance systems that anticipate—not lag behind—technological progress. It means embracing new tools, roles, and mindsets.
AI governance is no longer just a compliance requirement. It’s a foundational capability for scaling AI and growing your competitive advantage. The organisations that build it well will lead the next wave of intelligent transformation.
Because when AI starts thinking for itself, we’ll need to be even more thoughtful about how we govern it.
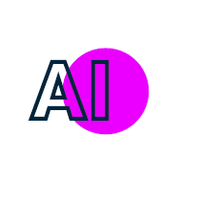
techUK - Seizing the AI Opportunity
For the UK to fully seize the AI opportunity, citizens and businesses must have trust and confidence in AI. techUK and our members champion the development of reliable and safe AI systems that align with the UK’s ethical principles.
AI assurance is central to this mission. Our members engage directly with policy makers, regulators, and industry leaders to influence policy and standards on AI safety and ethics, contributing to a responsible innovation environment. Through these efforts, we help build public trust in AI adoption whilst ensuring our members stay ahead of regulatory developments.
Get involved: techUK runs a busy calendar of activities including events, reports, and insights to demonstrate some of the most significant AI opportunities for the UK. Our AI Hub is where you will find details of all upcoming activity. We also send a monthly AI newsletter which you can subscribe to here.
Upcoming AI events
Latest news and insights
Subscribe to our AI newsletter
AI updates
Sign-up to our monthly newsletter to get the latest updates and opportunities from our AI and Data Analytics Programme straight to your inbox.
Contact the team
Visit our AI Hub - the home of all our AI content:
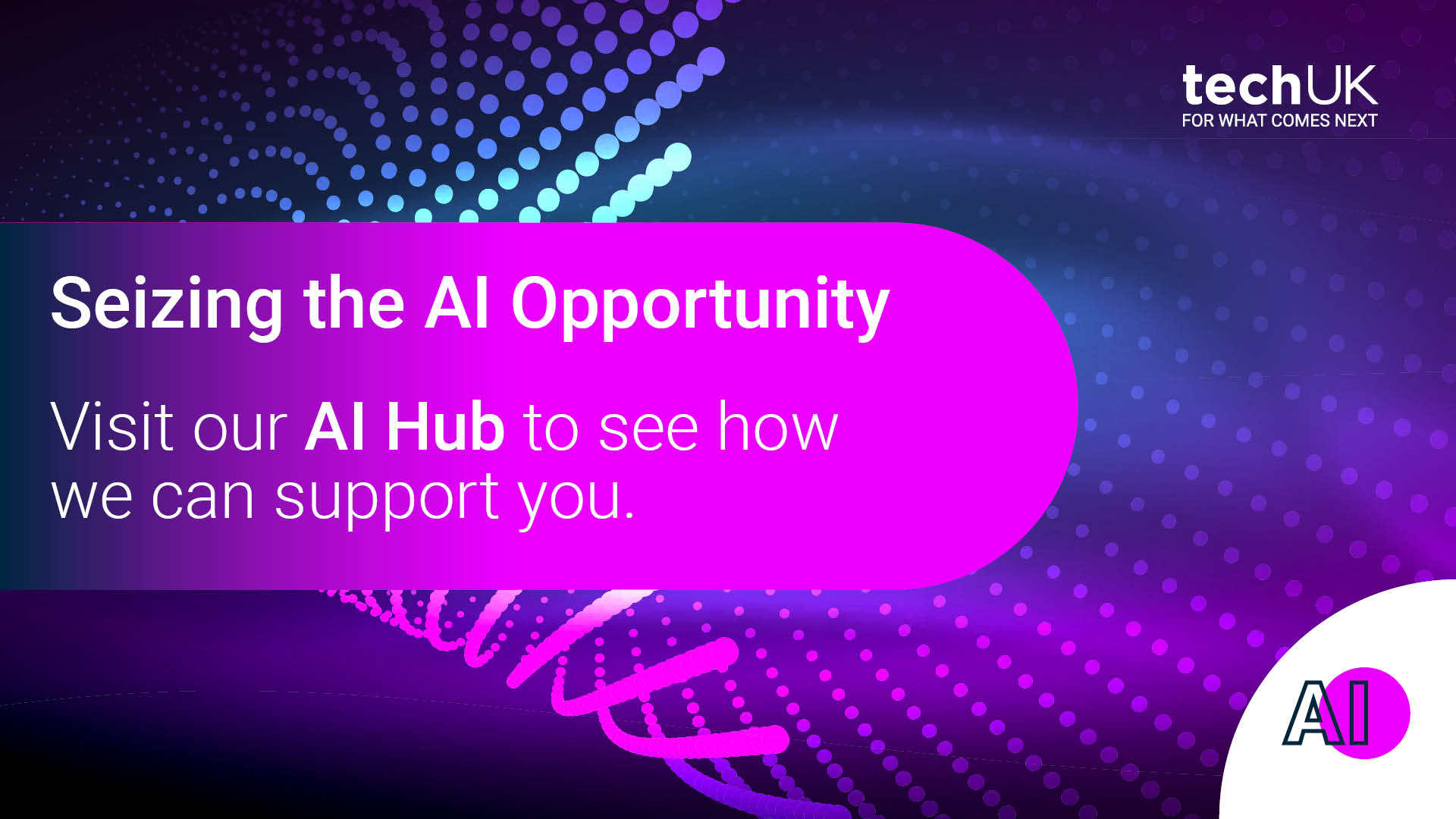