Guest blog (Blue Hat Associates): AI in action - revolutionising data structuring
AI retrieval technologies are transforming how organisations handle data, creating immense value from internal and external sources. It’s undoubtedly a game-changer when aligned with the right use cases. However, data quality issues (where source data has evolved over time and lacks standardisation) often hinder this potential, especially in data-driven sectors like healthcare.
A lesser-discussed use case, but crucial application of GenAI is iteratively improving an organisation's data "in flight”, lowering the cost and barriers for improving that data, its management and application of standards. This process, often referred to as “data structuring” or “information extraction”, uses various AI techniques to analyse, categorise and organise unstructured information from diverse sources.
The power of AI in data structuring
Natural Language Processing (NLP) and Machine Learning (ML) are helpful AI techniques for data structuring.
NLP algorithms can understand and interpret human language, allowing them to extract meaningful information from text-based sources such as emails, social media posts, customer reviews, and internal documents. These algorithms can identify entities, relationships, sentiment, and key topics within the text, forming the basis for structured data points that can then be served up as reports OR standardised into formal data structures and have taxonomies applied to align the data to an internal governance model.
ML models, particularly those using deep learning techniques, play a crucial role in recognising patterns and extracting insights from unstructured data. These models can be trained on large datasets to identify relevant information and categorise it according to predefined schemas or dynamically created structures. There are also huge amounts of available resources such as taxonomies and defined standards that can be added into these techniques aligning to internal governance with minimal user interaction.
Data structuring offers several benefits:
1. Data extraction: AI can automatically extract relevant data points from various unstructured sources using patterns in the data. For example, they can pull financial figures from earnings call transcripts, product specifications from technical documents, or customer sentiment from social media posts.
2. Data classification: AI can categorise unstructured data into predefined classes or create new categories based on content similarities. These classes can then be mapped to ontologies or taxonomies to identify preferred terms or add new synonyms. This helps in organising information for easier analysis and reporting.
3. Entity recognition: AI models can identify, and extract named entities such as people, organisations, locations and dates from unstructured text, creating structured data points for each entity type. Using taxonomy standards to enrich the training of these recognition systems also massively improves recall and precision.
4. Sentiment analysis: AI can analyse the emotional tone of text data, determining whether the sentiment is positive, negative, or neutral. This is particularly useful for customer feedback analysis and brand monitoring reports.
5. Topic modelling: AI algorithms can discover abstract topics within a collection of documents, helping to summarise large volumes of text data into key themes for reporting. This data can then be appropriately saved to be used as a larger “Knowledge Map” of the information to allow for the identification of broader trends and duplications.
6. Data validation and cleaning: AI can spot inconsistencies, errors or missing information in the extracted data, ensuring the quality and reliability of the structured output. This data can then be validated and fed back to the source systems (in an appropriate way that doesn’t destroy the integrity of the source) to improve quality.
7. Report generation: Once the data is structured, AI can automatically generate reports by selecting relevant data points, creating visualisations and even writing narrative summaries of the findings. Adding directives to structure the reports in a specific way or to add “companion metadata in say JSON format” allows these reports to become “golden sources” with the right governance.
8. Trend analysis: By processing large volumes of unstructured data over time, AI can identify trends and patterns that might be difficult for humans to detect, providing valuable insights for strategic decision-making.
9. Predictive analytics: Using historically structured data derived from unstructured sources, AI models can predict future trends or outcomes, enhancing the value of reports.
10. Multilingual processing: AI can process and structure data from sources in multiple languages, enabling global organisations to create comprehensive reports from geographically diverse data sources.
Creating opportunities for all types of organisations
By leveraging AI for data structuring, organisations of all sizes, can:
• Significantly reduce time and labour for data processing
• Handle larger volumes of information
• Improve consistency and reduce human error
• Enable more dynamic and real-time reporting
As new unstructured data becomes available, AI systems can continuously update structured databases and reports, providing up-to-date insights for decision-makers.
But remember, it’s important to keep a “human in the loop” with the AI acting as a companion or barrier reducer to remove the tedious parts of data standardisation with the human still being responsible for the final sign-off.
As AI technologies advance, we can expect even more sophisticated capabilities in transforming unstructured data. When deciding on an AI strategy, companies must consider both retrieval and enrichment of their data to fully leverage AI's potential.
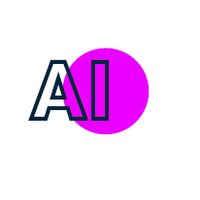
techUK - Seizing the AI Opportunity
The UK is a global leader in AI innovation, development and adoption.
The economic growth and productivity gain that AI can unlock is vast, but to fully harness this transformative opportunity, immediate action is required. Our aim is to ensure the UK seizes the opportunities presented by AI technology and continues to be a world leader in AI development.
Get involved: techUK runs a busy calendar of activities including events, reports, and insights to demonstrate some of the most significant AI opportunities for the UK. Our AI Hub is where you will find details of all upcoming activity. We also send a monthly AI newsletter which you can subscribe to here.
Upcoming AI events
Latest news and insights
Subscribe to our AI newsletter
AI and Data Analytics updates
Sign-up to our monthly newsletter to get the latest updates and opportunities from our AI and Data Analytics Programme straight to your inbox.
Contact the team
Visit our AI Hub - the home of all our AI content:
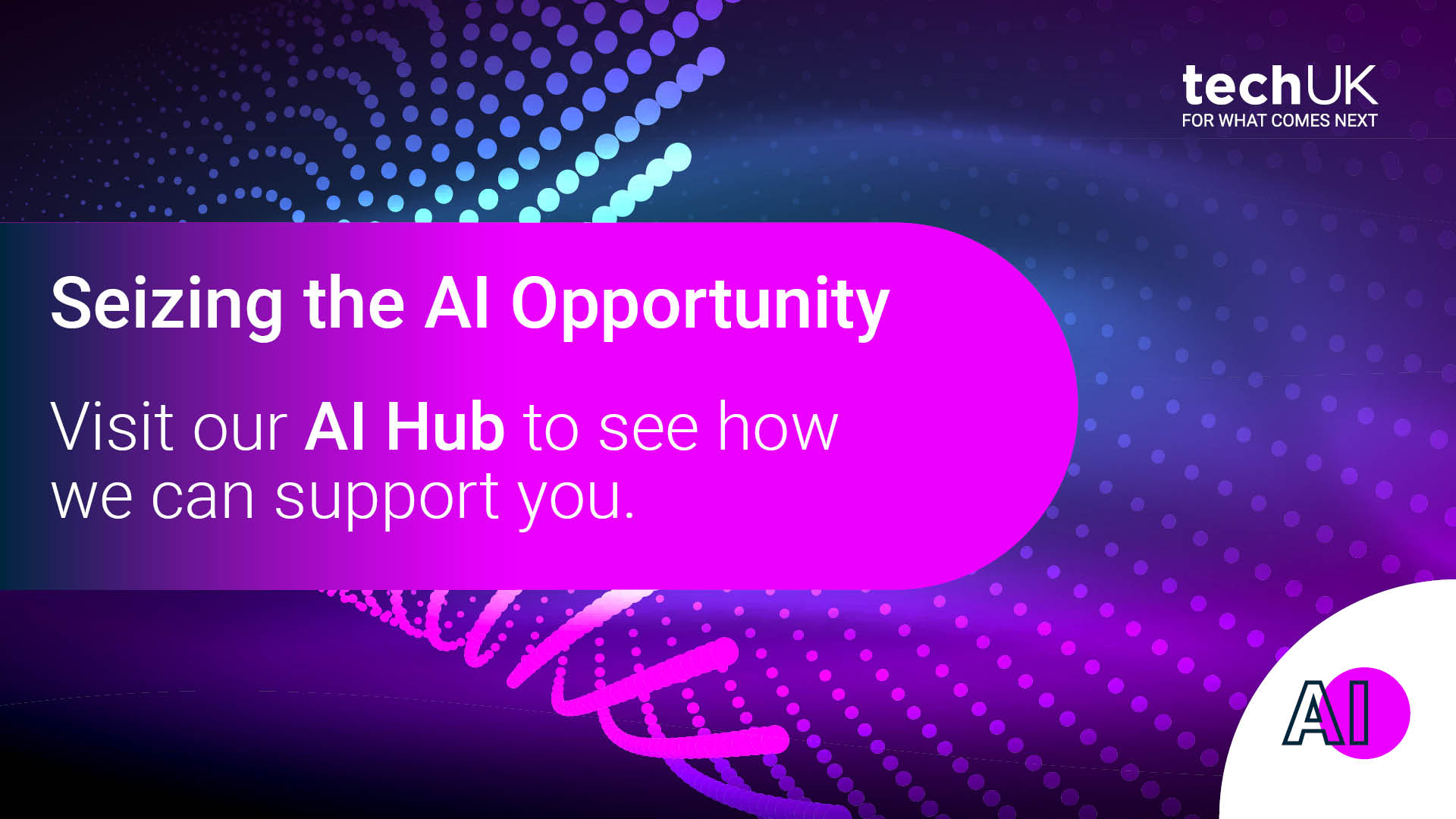