The Benevolent PlatformTM augments scientific ingenuity with AI to navigate an ever-expanding data landscape to map complex diseases (Guest blog by BenevolentAI)
Purpose: AI-enabled drug discovery to understand complex and underserved diseases
The primary use of AI at Benevolent is to understand complex disease biology, particularly in the context of a clinically-defined cohort of patients, to find new medicines for untreated and underserved diseases. To do so, we have built a robust data architecture and Knowledge Graph that captures a multidimensional representation of human biology across all diseases, which scientists can explore using our suite of AI predictive tools in the Benevolent Platform™. The tools reason across all of this information to suggest novel biological targets that could evolve into a drug discovery programme if experimentally validated, deemed safe and able to provide an opportunity to differentiate should the programme be successful.
At BenevolentAI, we invest much time in ingesting and harmonising a wide variety of biomedical data from more than 85 different data sources. We ingest pseudonymised or anonymised patient-level data, including electronic health records, genetic data, multi-omics data and scientific journals and literature. Our data is then represented in the form of a knowledge graph comprising nodes representing biological entities such as diseases, disease mechanisms, genes, cell types, and tissue types that are linked if they are related in some way.
BenevolentAI also uses large language models (LLM) to identify biological targets. Our models are trained to encode the meaning of literature sentences that refer to potential biological targets and are deployed to receive questions that ask for target suggestions for a particular disease, generate a ranked list of targets and provide evidence that backs up the target suggestions.
Barriers: Building trust in methodology and preventing algorithmic biases
One of the key barriers to AI deployment is trust in the methodology. Modern AI methods are typically black-box and provide limited rationale behind a prediction. As the typical user is unfamiliar with how the underpinning algorithms work, it is often difficult for them to act on a model suggestion, particularly when the supporting evidence they can understand (e.g. the literature) is sparse. The way to overcome these barriers is through investment in uncertainty quantification, explainable AI approaches and methods that look to identify causal relationships between objects of interest.
Another issue that we must continually be aware of is biases in data. Data bias can come in many forms. For example, biomedical literature is heavily biased towards a small number of diseases that are typically of interest to the developed world. We have to consider these biases when making predictions and have used data normalisation strategies to down-weight this type of information. Another source of data bias is at the patient level. Biobanks and other data sources provided by either Government or commercial organisations are heavily biased towards patients of European descent, with other ethnicities often underrepresented. We have developed tools to quantify diversity in data and have set up BenevolentAI’s Data Diversity Initiative to raise awareness of these issues.
Impact: Empowering scientists to discover drug targets for patients in need
Today, our Platform is scientifically and commercially validated. We have built an in-house pipeline of 15 named and 10 exploratory programmes, all Platform-generated, spanning multiple therapeutic areas in diseases affecting millions worldwide. Our most advanced asset is an atopic dermatitis topical compound in Phase 2a clinical development, and we also have a novel asset for ulcerative colitis planned to enter Phase 1 studies this year.
Secondly, our Platform supports a number of commercial and non-commercial partnerships, including a successful multi-year collaboration with AstraZeneca, who have already validated and selected a total of five novel targets identified by our Platform for their drug development portfolio in two disease areas: Idiopathic Pulmonary Fibrosis (IPF) & Chronic Kidney Disease (CKD).
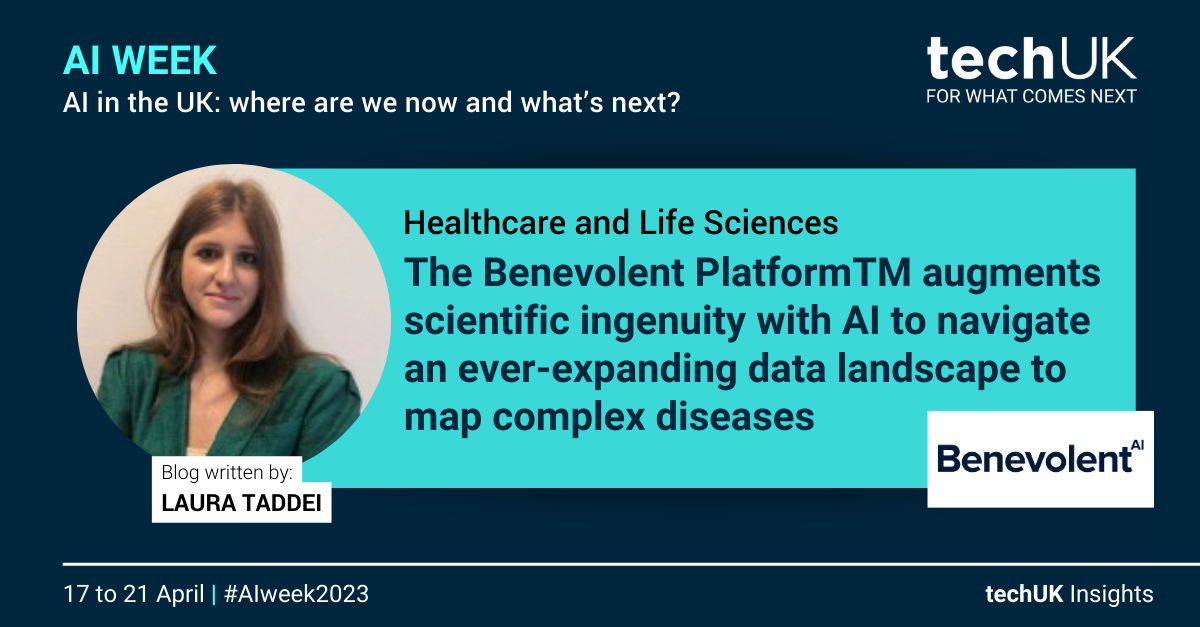
Get our tech and innovation insights straight to your inbox
Sign-up to get the latest updates and opportunities from our Technology and Innovation and AI programmes.